Human Vehicle Interaction
Our group works with biometric evaluation and modelling of driver behaviour in vehicles, specifically in reference to self-driving cars. We seek to develop insights into patterns of biometric activity and behaviour that are correlated with particular affective states. For example, we are interested in how facial expression, galvanic skin response, eye movements, driver pose/distance from wheel, braking and steering behaviour, correlate with feelings of comfort/discomfort when driving which might provide cues to systems used in conjunction automated emergency braking.
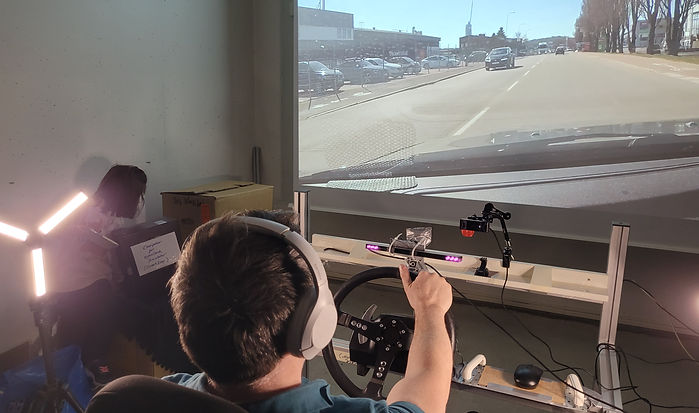
How we work with Human Vehicle Interaction
At DICE Lab we are investigating scenarios in car simulator setups for assessing how humans (drivers, passengers) respond affectively and behaviourally in potentially dangerous driving scenarios for the purpose of using such information to automate corrective responses in self-driving cars. Currently the target is for use in cars at around SAE level 2, e.g. where automated emergency braking may be utilized. We (Robert Lowe at RISE – Research Institutes of Sweden, Humanized Autonomy Unit) is also working on research into how to use Explainable AI (XAI) within the Machine Learning development life cycle for autonomous vehicles.
Current Projects
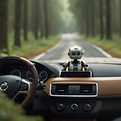
I-AIMS (Impairment-Aware Intelligent Mobility System)
I-AIMS aims at investigating how to enhance safety and well-being of drivers through i) monitoring, and ii) regulating impaired cognitive and emotional states affected by driving scenarios and/or driver state. The University of Gothenburg (including personnel at DICE Lab) are collaborating with SmartEye in using Smart-Eye’s Driver Monitoring System (DMS) focusing on eye-tracking cameras for sensing e.g. stress levels and cognitive load. We are investigating how the use of a large language model (LLM) can regulate affective state following feedback from the DMS.
In the longer term, we plan to develop the LLM interface to the DMS by allowing algorithmic supervision of the LLM output that is task and context appropriate and to incorporate embodied feedback in the form of a small (robot) head unit. This unit will display emotional expressions and have two degrees of freedom for providing interactions with drivers at opportune (safe) moments.
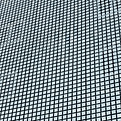
EFFECT (Efficient human-centered safety systems)
The aim of the project is to develop a computationally efficient ADAS, in the form of an automated emergency braking system (AEB) for cars interacting with different vehicles in scenarios that are potentially safety critical. A research focus is on the concept of driver “comfort zone boundaries” whereby driver (or passenger) discomfort is assessed in scenarios that have the potential to be, but are not yet, safety-critical (requiring AEB). Our group’s focus is on development of Deep Learning based algorithms for detecting driver state to assess such comfort zone boundaries.
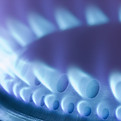
SAFEXPLAIN (Safe and Explainable Critical Embedded Systems Based on AI)
The aim of the project is to address the functional safety requirements of Critical Autonomous AI-Based Systems (CAIS) by making more transparent (explainable, traceable) the black box algorithms (typically Deep Learning based) used/to-be-used within software products for autonomous vehicles. The focus of RISE AB (within which Robert Lowe of DICE Lab works) is to identify and deploy Explainable AI (XAI) algorithms at different stages of the Machine Learning lifecycle to render more transparent the black box algorithms for the various users involved (e.g. developers, operational users). Read more about the project here